기사본문
Lunit’s Chief Medical Officer Unveils ‘AI Biomarker’: The Four Key Needs of Pharma
입력 2024-08-20 08:38 수정 2024-08-20 22:40
by Sungmin Kim
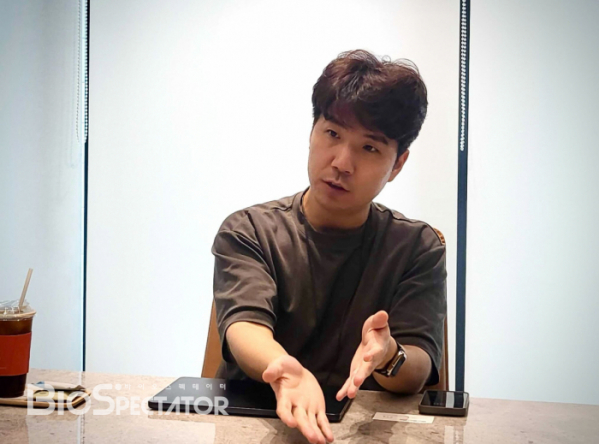
▲Interview with Chan-Young Ock, Chief Medical Officer (CMO) of Lunit, and BioSpectator
“Pathological biomarkers” which are the standard for prescribing anticancer drugs in artificial intelligence (AI) are being redefined. Until now, biomarker analysis technology and clinical application in the field have often failed to keep up with the pace of development of anticancer drugs. Because of this gap, biomarkers have been recognized as important and necessary, but in clinical development and in the clinic, they have been viewed as a limiting factor in the treatment of patients.
However, recently, as AI has begun to intervene in biomarker analysis, the situation is changing. Similar changes appear to be taking place in cancer, as if the advent of the Hubble telescope, which can observe distant space, opened the age of astronomy. As AI is now able to analyze cancer tissue biomarkers on a cell-by-bell basis, which was previously identified by the eyes of pathologists, we are now entering a phase where technology can advance science. At the same time, over the past three to four years, as technologies that directly target cancer cells such as antibody-drug conjugates (ADCs) and radiopharmaceutical therapies(RPTs) have matured, biomarker issues are becoming more prominent in clinical development and medical field settings.
Chan-Young Ock, Chief Medical Officer (CMO) of Lunit, reunited with Biospectator again after 2 years and said, "When I joined Lunit five years ago, I started with the the idea that pathology data could be meaningful in the field of anticancer drugs. No one gave us an answer, but we decided that we could bring enough value when we thought about it ourselves,” he said. "Even two or three years ago, Big Pharma companies tried to organize an internal team to drive it, but now they have completely turned (to external partnerships). The part that global pharmaceutical companies see as very important is commercial readiness, and we are currently discussing a contract with CLIA lab in the United States and it is expected to be established soon," he said.
Lunit has been developing ‘Lunit SCOPE’, an AI biomarker platform that predicts response of anticancer drugs, into four areas at global academic conferences and has accumulated evidence by publishing 106 academic conferences and 27 papers. It has been recognized as one of the top three globally in a bake-off where it was directly compared to its competitors. It's definitely the way to go, and now it's turning into a battle of who Big Pharma will go with. This is also reflected in the number of requests for research and analysis from global pharma companies to Lunit SCOPE. The number of their requests to Lunit SCOPE began to increase late last year and surpassed 5,000 as of July, compared to 1,000 last year.
However, it’s worth breaking down that 5,000 number even further. This is because AI-powered biomarkers are creating a much broader, more fundamental shift in pathology. So far, Lunits has expanded Lunit SCOPE to four different areas, each of which Big Pharma has different needs.
Lunit sees the current situation as similar to the time when AI introduced to radiology four to five years ago. Once the value of AI in radiology was recognized, numerous competitors jumped in, and Lunit signed partnerships with global companies such as Fujifilm, GE Healthcare, and Phillips on “Lunit INSIGHT." Since then, the wave has passed, and only a few leading groups, including Lunit, are left.
The near future of AI's expansion in the medical field is likely to be AI biomarkers that help prescribe drugs in complex pathological cancer tissue images, and the next two to three years will be crucial for Lunit. On this day, CMO Ock Chan-young introduced the development status of Lunit SCOPE, a drug response prediction platform, including the application areas of four platforms: ▲ immuno-oncology(IO), ▲universal immunohistochemical tissue staining (Universal IHC, UIHC), ▲ genotype predictor for screening cancer gene mutations, ▲ CT image-based drug response prediction, and positioning according to the needs of Big Pharma.
Needs ① ‘Prospective clinical’ application of immuno-oncology drugs...'FDA/insurance company' needs
Of the 5,000 requests for research analytics from global pharmaceutical companies, approximately 80% (over 4,000) were for Lunit to apply ‘Lunit SCOPE IO’ to predict immune-oncology response. Lunit SCOPE IO is the first AI biomarker platform developed by Lunit, and is a concept that quantitatively analyzes immune phenotyping based on the number, density, and distribution of immune cells in the tumor microenvironment (TME) in H&E-stained images. It can use existing H&E images without additional tissue staining, so it can be analyzed within the pathologist's workflow without tissue loss.
Dr. Ock said, "With PD-1 and PD-L1 immuno-oncology drugs now being prescribed across nearly all caners, understanding the tumor immune landscape is becoming increasingly important as we consider what additional drugs to give. Big Pharma will use Lunit SCOPE IO for two main purposes in clinical development of immuno-oncology drugs."
First, the purpose is to objectively assess biomarkers. "When comparing cancer patients before and after the administration of immuno-oncology drug candidates, we will analyze whether the drug worked as the expected mechanism of action (MoA), such as few immune cells in the tumor tissue and an increase in immune cells after treatment, and whether this phenomenon is associated with the patient’s outcome,” said Ock, explaining that this is the area where we receive the most requests.
Furthermore, the team will evaluate whether in-depth analysis of a patient’s cancer tissue prior to treatment can predict subsequent outcomes with immune-oncology drugs. “For pharmaceutical companies, identifying patients who will respond to an immune-oncology candidate is the key to good outcomes in clinical trials, and without a pre-screening tool, clinical development is more likely to fail,” said Ock. "As clinical trials get larger and more expensive, it is imperative to exclude patients who will not respond and include those who will.”
To date, we have received over 4,000 requests from global pharmaceutical companies to provide data from completed immune-oncology trials, to see if we can find meaningful biomarkers, and to see if we can predict drug response by looking at pre-treatment tissue. The results are being discussed further. “The next step is to apply the biomarkers to a failed clinical data set to drive re-licensure or, now that we have confirmed feasibility, to collaborate on applying the biomarkers to a prospective clinical trial of an immune-oncology drug,” says Ock.
Over the past year, Lunit SCOPE IO has expanded from looking at the spatial distribution of stroma to looking at the function of specific cells, such as macrophages, fibroblasts, and endothelial cells. Each of these are factors that can influence the activity of immuno-oncology drugs.
For example, the TGF-β system is a major signaling pathway for drug resistance to immuno-oncology drugs. In colorectal and pancreatic cancer, TGF-β activation increases the number of fibroblasts in the tumor and over-activates them, creating a thick matrix in the tumor microenvironment that prevents immune cells from entering. The concept is to overcome drug resistance with TGF- β inhibitors. However, so far, TGF- β inhibitors have not shown clear efficacy in the clinical stage, which is where the need for biomarkers comes in.
"Recently, TGF-β inhibitors are being developed again, and if you look at the global competitive landscape, there are about three big pharma companies developing them," said Ock. "We are discussing with one of them about applying Lunit's AI biomarker from the phase 2 stage, and now there is a greater need from the Big Pharma side to say, ‘We can’t do without biomarkers."
Another area to keep an eye on is the growing needs of regulators and payers. “Pharma’s position may be different from that of the U.S. Food and Drug Administration (FDA), insurance companies, and researchers," said Ock. "It is important for Pharma to increase the number of markets in which they can sell their drugs, but it’s not the same for the regulators or doctors who pay for the drugs and prescribe them to patients. We wonder whether the drug is really helpful to the patient or whether there is a better treatment option, whether it is not harmful to the patient, and whether the treatment option is economical," he said.
In other words, Pharma is interested in biomarkers that increase the number of patients to prescribe a drug to, while regulators, insurers, and researchers are interested in excluding patients who do not respond to drugs. This is where biomarkers can intervene.
Lunit is interested in the phenomenon of hyper-progression, where the PD-(L)1 immuno-oncology drug is widely prescribed to patients, but the disease progresses rapidly after the drug is administered, to the detriment of the patient. In this case, Lunit is looking for a biomarker that can pre-screen patients who will have a worse prognosis after receiving immune-oncology drugs.
While the FDA has historically favored approving PD-(L)1 immuno-oncology drug with additional agents and expanded indications, the agency recently issued dose optimization guidance to ensure that patients do not receive higher doses than necessary, even at the time of approval. In addition, the FDA is taking a hard line on developers, discouraging them from adding unnecessary agents to combinations of anti-cancer drugs.
"More recently, the FDA and insurers have been looking for models to make patient care safer, and the FDA has specifically told us to bring in models that can reduce unnecessary prescriptions,” Ock said. “From an insurer’s perspective, if we can exclude patients who are not responding to a drug by a certain biomarker rather than giving them more drugs, it makes sense to pay for it.”
Along the same lines is Oncotype DX (Oncotype DX), a breast cancer diagnostic. It analyzes the activity of 21 genes in breast cancer tissue to determine a breast cancer patient’s response to chemotherapy and the likelihood of recurrence, which helps determine the right treatment option for the patient. The results can lead to decisions such as adding chemotherapy and hormone therapy or excluding chemotherapy. From an insurer’s perspective, it makes sense to spend money on biomarkers that can guide cancer treatment compared to paying for drugs.
Needs ② ADC/RPT emergence...cancer antigen 'tumor, cell membrane' specific expression of " issue"
Next is Lunit's Universal Immunohistochemical Histochemistry (UIHC), which uses AI technology to quantitatively analyze the amount of protein expression expressed by tumor cells in IHC images. It quantitatively presents the expression of proteins such as HER2, TROP2, and Claudin 18.2 (CLDN 18.2) expressed by tumor cells in IHC images.
"Over the past two to three years, there has been a tremendous amount of interest in how to target cancer antigens that are selectively expressed on the membrane of cancer cells with breakthroughs in ADC,” said Ock. “More recently, there have been radiopharmaceuticals (RPTs), which are powerful tools to attack cancer cells by attaching radioactive material to antibodies. With advances in technology, it is now not difficult to attack cancer cells if you find the right target.”
With this shift in cancer treatment modalities comes a need for Big Pharma. “There are two big questions,” says Ock. “They want to know if the target of interest is actually expressed primarily in cancer cells and not in normal cells, and if the target is really expressed on the surface of the cell membrane in cancer cells and not in the nucleus or elsewhere,” explains Ock.
Until now, it was an area that pathologists used to determine by tumor microarray (TMA) analysis. TMA involves taking dozens of punches of small circles from cancer tissues at a time, placing them on a slide, and analyzing them. One circle (core) contains 10,000 to 100,000 tumor cells, and you usually get 30 cores at a time. If you make 7 slides, you get about 200 cores. The pathologist looks at these 200 stained cores, looks at the expression pattern, and gives a reading such as IHC1+ or IHC3+.
However, this approach is bound to be subjective. It's something AI can do better, and Big Pharma simply wants more detailed data than AI quantifying its expression criteria. "Pharma wants values for each of the tens of thousands of cells in its core,” said Ock. " They want to read the cellular level information to see whether the cancer antigen is expressed on cancer cells or other cells, and to know whether it is expressed precisely on the cell membrane or in non-specific areas,” he said.
This is because the side effects of drugs acting on normal cells in clinical practice are becoming a problem as the drug's aggression grows against the background of the growing needs of Big Pharma. HER2 ADC "Enhertu (DX-d)" has had encouraging clinical effects, but interstitial lung disease (ILD) and pneumonia are becoming an issue, and the FDA label states that if there are side effects of grade 2 or higher, the drug should be permanently discontinued.
As the development of radiopharmaceuticals is in full swing, side effects are becoming an issue. As J&J moves to develop next-generation PSMA radiopharmaceuticals in prostate cancer, the company raised concerns at the American Society of Clinical Oncology (ASCO) this year when it announced that four patients died due to side effects of drug administration in the first-in-human Phase 1 clinical trial of JNJ-6420, a KLK2 target actinium-225 (225Ac) in prostate cancer.
Furthermore, Lunit is conducting AI analysis of 7 million core information in an open database (DB), and can provide critical information such as “contrary to expectations, the target antigen is expressed in normal tissues. It is also possible to discover new cancer antigens that are suitable for developing ADCs or RPTs that were previously unknown in an unbiased way. Of the 30,000 proteins in the body, only about 100 cancer antigens have been identified by researchers as specific to cancer cells and cell membranes.
At this stage, global pharmaceutical companies developing ADCs or RPTs are commissioning UIHC assays to validate their targets before entering clinical development. As a potential companion diagnostic (CDx) biomarker in the next phase of clinical development, AI analysis can provide not only IHC values (0/1+/2+/3+) but also specific information about whether the tumor antigen is expressed on the cancer cells, located on the membrane, etc.
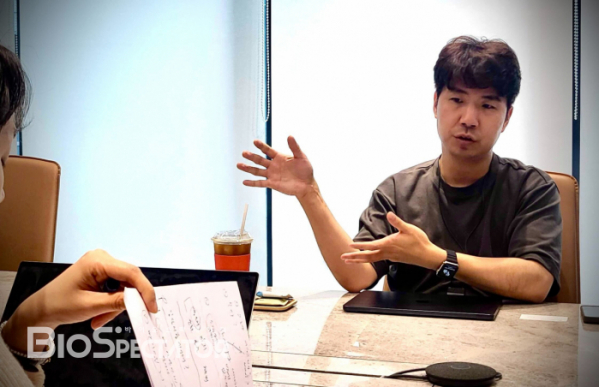
▲Interview with Chan-Young Ock, Chief Medical Officer (CMO) of Lunit, and BioSpectator
Needs ③ 3 'Genetic alteration prediction' in H&E..."Pre-screening Tool"
Lunit's genotype predictor is a model that uses AI to predict the presence of multiple gene alterations in cancer tissue H&E images, including EGFR, KRAS, ALK, ROS1, RET, and MET. Although it was developed later than other AI biomarker models, the platform is gaining attraction among global pharmaceutical companies.
At first glance, it may seem puzzling to predict genetic alterations based on tissue images alone, but the assumption is that the gene alterations have affected the expression of specific proteins. The assumption is that the AI can pick up on subtle changes in histology that the human eye can’t see. We don't know what information AI is looking at specifically. The AI is designed to learn from images and match specific variants, regardless of the genetic mutation type, and this is where it can continue to scale.
These genotype predicators are intended to ‘pre-screen’ before testing or NGS for specific genetic alterations for treatment decisions. It’s easy to see how this could be a real-world problem in clinical development or therapy.
When biopsy is performed in patients with non-small cell lung cancer, tissue is usually obtained from 5 to 10 pieces. After performing basic H&E, EGFR (PCR), ALK (IHC, FISH), and PD-L1 (IHC) tests, only a few tissues are left, and the remaining tissues that can be treated with targeted antitumor drugs have mutations such as ROS1, RET, and MET, but they are expressed at low frequencies. At this point, there may not be enough tissue for NGS, and even if there is, it costs $3,500 and takes 3 weeks to receive the results.
This can lead to commercialization difficulties. Last year, Roche returned its RET inhibitor Gavreto (pralsetinib), which it bought from Blueprint Medicines for $775 million in upfront payments alone. Roche had originally purchased Gavreto based on high hopes for the biomarker-based cancer drug, but later decided to discontinue the drug to cut losses following poor sales. RET mutations are rare in NSCLc, with an incidence of 1-2%.
The situation is the same in clinical development, and there are two main aspects of what global pharmaceutical companies are thirsty for. The first is to increase clinical efficiency, especially in drug development targeting rare mutations. Ock said, "If you have a variation that is expressed at about 5% in all cancer patients or specific carcinomas, 95 patients are excluded from clinical practice when NGS is performed on 100 patients. For Pharma, that’s a lot of money, and more importantly, it slows down clinical progress. It is currently a very important agenda in terms of clinical trial efficiency.”
"If our AI narrows down the number of patients who will have mutations and can reduce it to only 20 people for NGS, excluding patients without mutations, efficiency in terms of speed and cost can be improved four times," he said. "So, the 20 billion won to 30 billion won that’s spent on these platforms as a prescreening tool for clinical trials is not even close, and we’re talking to two global pharmaceutical companies, one of which is finalizing due diligence and talking about the next steps.”
Second, when Big Pharma develops clinical development, it can use the remaining tissue more efficiently. For Pharma, cancer tissue is a very valuable research resource. If Lunit's Genotype Predictor provides information on what characteristics the tissue has in the H&E image in the workflow without loss of tissue, it can help narrow down Pharma's concerns and options.
It can also help doctors make choices in the medical field. In the real world, getting an NGS test or liquid biopsy for a rare mutation is expensive and takes 2 to 3 weeks. This is why doctors often prescribe treatments that can be prescribed without biomarkers, and Lunit’s Genotype Predictor can help doctors and patients get better drugs with additional testing by showing that certain biomarkers are likely to be present.
“This concept can be expanded in the future to predict protein expression as well as genetic mutations,” said Ock. “With new drugs coming out all the time, including ADCs, it’s important to give patients some direction when they are first diagnosed."
Needs ④ Expanding to 'drug response prediction' with radiology CT
Lunit is expanding the scope of AI biomarkers for cancer to include AI models that predict immune-oncology drug responsiveness based on CT image data.
The tumor microenvironment in the tissue, which is what Lunit focused on first, is heterogeneous and dynamically changing in nature. CT images, on the other hand, provide information about the distribution, number, and shape of tumors, as well as the surrounding normal lung features, at a more macro-level. Ock joined Lunit not only because he wanted to make the most of the information in pathology tissues using AI tools, but also because, as a former oncologist, he thought it would be meaningful to apply AI to the differences he intuitively noticed in patients’ CT images.
Lunit has begun to showcase this potential, presenting a study at this year’s American Society of Clinical Oncology (ASCO 2024) that showed its AI model could predict immune-oncology drug response based on chest CT data in patients with NSCLC. The deep learning CT model predicted immune-oncology drug response independently of traditional Lunit Insights and provided additional information when both CT and tissue biomarker data were used.
"Big Pharma saw this data and commented that the biggest difference between Lunit and the other two or three AI pathology competitors is that we’re also applying AI in radiology,” said Ock, adding that he thinks Lunit will be the fastest to adapt if its capabilities in radiology can also be used to analyze biomarkers that predict drug response in cancer patients. The application of CT results to clinical trials is also being discussed.
"Now we're going into the pharma business phase...Start setting up CLIA labs"
Lunit’s ultimate goal with its pathology AI biomarker is to get FDA clearance as a companion diagnostic (CDx) biomarker for prescribing cancer drugs. However, for pathology diagnostics, there is a pathway to commercial dissemination as a complementary biomarker by setting up a CLIA lab. It is a structure that can be paid by pharmaceutical companies' clinical development, physicians, and insurance companies (payers).
Ock divided it into three stages. First, the company is actively contracting to set up a CLIA lab for a reputable CRO in the U.S., which is expected to be finalized soon. “For global pharma, it’s very important to be commercially ready,” he says. “The performance of the biomarker is a prerequisite, and we’ve been asked very specifically if we have a plan for the next step, which is to set up a CLIA lab. We don’t necessarily think that a biomarker has to be a perfect 100, but rather, if it’s a 70 or 80, but it’s in circulation and it’s actually successful in the clinic, it’s important that the system is set up so that hospitals and patients can use it.”
The next step will be to retrospectively analyze clinical data from Pharma’s previous failed or ambiguous trials to identify meaningful AI biomarkers to drive prospective clinical development. Finally, Lunit’s AI biomarkers will be validated in prospective clinical trials and approved. In both cases, pharmaceutical companies will pay Lunit for research on the use of biomarkers.
Finally, Ock concluded, "The agenda we set out when we joined Lunit five years ago is being realized. Two to three years ago, Big Pharma did not seem to believe in Lunit SCOPE IO, but now it is proposing a prospective clinical trial, and UIHC is also emerging as the ADC market opens. "We are also encouraged that the results of the gene mutation prediction AI model and CT model are coming together so far," he said, expressing expectations for the future.
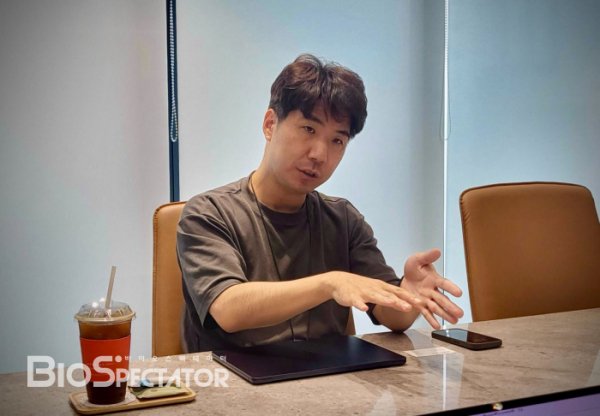
▲Interview with Chan-Young Ock, Chief Medical Officer (CMO) of Lunit, and BioSpectator