기사본문
Lunit, Combining AI improves radiologists' readings of mammograms
입력 2020-03-05 09:40 수정 2020-03-05 09:40
바이오스펙테이터 Sungmin Kim 기자
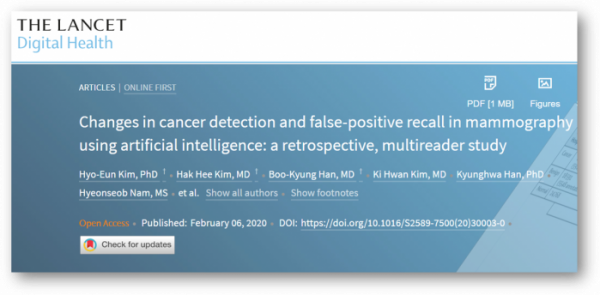
It was reported in the research that artificial intelligence (AI) can improve the efficiency of conventional cancer screening reading. In the case employing AI for the diagnosis of breast cancer by radiologist, the sensitivity, in finding patients of breast cancer, increased from 75.3% to 84.8%. Besides, the forms of breast cancer which were difficult to find by the radiologist, together with the positive consequences of higher probability in finding breast cancer, were identified with the employment of AI.
Lunit, the representative domestic enterprise of medical artificial intelligence, announced on the 10th that the company published the result of research of an improved accuracy in the reading of conventional breast cancer by exploiting the artificial intelligence developed spontaneously in ‘The Lancet Digital Health’ on the 6th (10.1016/S2589-7500(20)30003-0).
The outcomes resulted from the analysis of over 170,000 data collected from the five domestic medical institutions including Severance Hospital of Yonsei University, Asan Medical Center, and Samsung Medical Center etc., and other overseas institutions in America and in the United Kingdom, as well as other institutions.
The significance of results of research can be found from the biggest dataset of breast cancer employed for the study, among studies of ‘AI Diagnosis of Breast Cancer’ which had been ever conducted. Currently, the mammography test is frequently used for the screening of patients of breast cancer. The mammography enables us to find patients suffering breast cancer earlier, thereby reducing the incidence of mortality for those patients. However, 10-30% of patients suffering breast cancer are diagnosed with a false-negative result, due to lesions of breast cancer hidden by dense parenchyma, or errors in recognition/reading. That is, the lesions of cancer are not found properly.
Besides, approximately 28.6% of patients among those diagnosed with positive breast cancer via mammography are definitely diagnosed with breast cancer in practice (USA, 2017). The difficulty in the reading of mammographic images also contributes to the differed accuracies of diagnoses of breast cancer. In regard to the sensitivity of reading, it has been know that it varies in the range up to maximum 20%. Thus, Lunit has raised the accuracy of reading of mammographic images to find patients suffering breast cancer, and developed the AI based auxiliary software for the diagnosis of breast cancer capable of reducing the number of unnecessary examinations.
The results of research, published in the journal of Lancet, are differentiated in terms of three aspects compared to the computer-aided detection (CAD) technology employed in the mammography. First, Lunit utilized the large scaled quality data set for the development of the product, particularly based on the biggest number of malicious samples. Second, the research proceeded as the multi-centered one comparing to previous research conducted by a single or two institutions. Third, the research exploited the multi-ethnical data (Asian and White) collected from several countries, compared to previously conducted research.
Researchers in the Lunit exploited a total of 145,663 samples of mammographic images comprising 36,468 samples obtained through the biopsy (histological examination) of patients definitely diagnosed with breast cancer, 59,544 samples obtained through biopsy (histological examination) from patients diagnosed with benign tumor, and 74,812 samples obtained from normal patients, for the training of AI and development of new product. Only one sample collected from each patient was used. The entire data set consisted of 145,663 samples of Korean, 18,024 samples of American, and 6,543 samples of English.
The company expatiated, “… over 36,000 images of breast definitely diagnosed with breast cancer through biopsy were employed for the development of new product; the size of data set was the biggest one among global AI researches exploiting mammography…”. The number of samples used for the development of CAD employing mammography was less than 5,000 (Pubmed 2020.01).
Lunit designed the research, and collected the data set from several institutions to train the artificial intelligence (AI) for deep learning. The research team concluded the ‘deep learning’ would be more appropriate than ‘machine learning’ mediated by human in the learning process for the training, in terms of the difference in accuracy of diagnosis conducted by a radiologist. Thereafter, the AI algorithm was developed by using the CNN model selected among models of deep learning together with the auxiliary diagnostic software for breast cancer.
Now, let’s take a look at key results of the research published in the journal of Lancet. In conclusion, the accuracy of discriminating breast cancer through mammographic images has improved with the use of AI by a radiologist. Here 14 radiologists have participated in the research and read a total of 320 sample images comprising 160 samples of cancer and 160 samples of normal ones.
Above all, in respect of the accuracy of detecting breast cancer, the AI marked 88.8% (82.80-93.19) of sensitivity whereas the radiologists exhibited 75.3% (73.43-77.04) thereof. And the cases of radiologists assisted by AI rendered the sensitivity increased to 84.8% (83.22-86.24). Besides, in terms of the indicator of specificity signifying the capability of excluding patients other than breast cancer, the AI marked 81.7% (75.02-87.51) of specificity, while the radiologists exhibited 71.96% (70.05-73.82) thereof. The radiologists, exploited the assistance of AI, manifested the specificity increased to 74.64% (72.29-76.43).
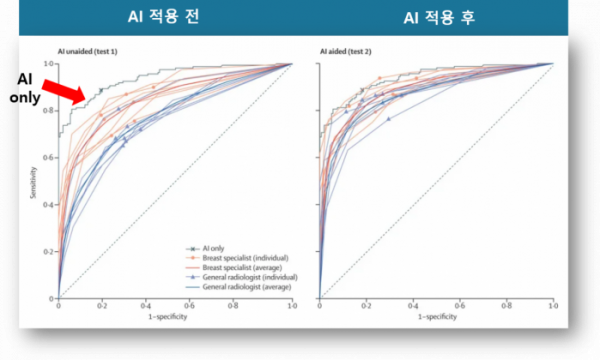
What are the reasons behind the improvement in accuracy of reading by the use of AI? The research members explained that the AI exhibited higher accuracy in detecting certain types of breast cancers compared to the radiologists; the details are also explained broadly in cases of three aspects as follows.
First, the AI rendered higher probability of detecting mass as compared to radiologists (90% vs 78%, p=0.044) in the results of research, and proved its sensitivity of detecting distorted or asymmetrical breast cancer superior to existing AI made for diagnosis of breast cancer (90% vs 50%, p=0.023). This can secure the competitive advantage of definitely using AI for diagnosis purposes.
Second, the higher accuracy of AI exhibited an excellent performance in the detection of early infiltrative breast cancer which is difficult to diagnose. Contrary to this the radiologists exhibited 74% of sensitivities of detection commonly for T1 cancer and node-negative cancers in lymph nodes, the AI exhibited sensitivities of 91% and 87%, respectively (p=0.039 and p=0.0025, respectively).
Third, the diagnostic performance of AI was affected relatively less by the density of breast. The density of breast tissue is a key factor for the diagnosis of mammographic images. Asian people typically show higher ratio of dense breast than those of Western people resulting in the higher probability of hiding lesions of cancer by dense tissues of breast causing the decreasing accuracy of reading mammographic images to find breast cancer. According to previous papers, the sensitivity of doctors corresponding to dense breast was 73.1% comparing to 79.2% corresponded to non-dense breast. And the sensitivity of detecting breast cancer of dense breast by doctors assisted by AI improved significantly to 85.0%.
Director Kim Hyo-eun, the CPO of Lunit and also is the first coauthor of the paper published in the journal of Lancet, explained, “…characteristics of breast density varies according to ethnic background, and the accuracy of detection of breast cancer also varies according to environment of capturing images and photographic equipment. Thus, the integration of algorithm spontaneously developed for past 4 years with the quality data set that taken the characteristics into account rendered the significant results…”.
Professor Kim Eun-gyeong of the medical college in Yonsei University who was in charge of the research stated, “… one of great difficulties in reading mammographic images is the additional examination to be conducted with the intention of reducing lesions missed in the reading of mammographic images most of which would result in unnecessary ones…”, “… according to our study, the artificial intelligence helped us to find more breast cancers without the increase of false positive recall rate. Above all, the possibility of finding early breast cancer was identified…”.
The research was conducted retrospectively and the accumulation of real-world data is expected to continue in accordance with the development of product. In the meantime, Lunit acquired an approval of the MMG (Lunit INSIGHT for Mammography) as an Auxiliary Software of the Third Class for the diagnosis of breast cancer from the Ministry of Food and Drug Safety last year.