기사본문
Lunit’s ‘expansion’, ‘TIL biomarker’ and its strategy for PD-1 market
입력 2022-08-17 10:59 수정 2022-08-17 10:59
by Sungmin Kim
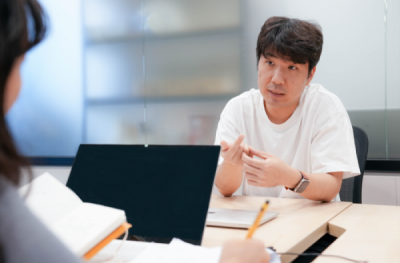
▲Chan-young Ock, chief medical officer
Lunit, which has established a foundation as a global medical AI diagnostics company, is planning to penetrate the 50% of the market, where immune checkpoint inhibitors are not prescribed, with a new AI biomarker that quantifies tumor-infiltrating lymphocytes (TIL) in cancer tissues. Competition to develop PD-1 and PD-L1 immune checkpoint inhibitor is currently in progress. Although immune checkpoint inhibitors are prescribed for about half of all cancers in eight years after their first launch, half of the patients still do not receive the benefits of checkpoint inhibitors.
The keyword Lunit is promoting is an AI biomarker that predicts drug response within pathological tissue (cancer tissue of the patient). There is still no AI biomarker in the field of therapeutics yet. It is easy to think of it this way. MSD, the world's top pharmaceutical and the developer of the PD-1 antibody 'Keytruda', which is widening a gap with latecomers in the immune checkpoint inhibitor market, is one of the Big Pharma that understands biomarkers best. In terms of biomarkers, Keytruda is largely prescribed for 5 PD-L1 positive solid carcinomas. Taking this one step further, Keytruda is the only immunotherapy that can be prescribed with both biomarkers, MSI-H/dMMR (approved in 2017) or tumor mutation burden (TMB, approved in 2020), regardless of cancer types. Also called tissue-agnostic therapy.
The strategy that Lunit benchmarks is ultimately to find patients who can be prescribed for immuno-cancer drugs based on "inflamed immune phenotype (IIP)" biomarkers in cancer tissues with AI regardless of cancer. It is an AI biomarker with the concept of companion diagnostics (CDx). In this respect, this year is also an important year for Lunit to expand from imaging diagnosis to therapeutic diagnosis.
As a starting point, Lunit announced to JCO this year the potential of AI biomarkers as biomarkers that can predict the response rate of checkpoint inhibitors in lung cancer regardless of PD-L1 expression. Then, at the American Society of Clinical Oncology (ASCO) in June, an initial study result was presented that IIP biomarkers can predict immune checkpoint inhibitor drug response from lung cancer to 16 carcinomas.
A movement to another axis has also begun; development of biomarkers for specific protein expression in cancer tissues with AI. The highlight of this year's ASCO was the encouraging results of AstraZeneca and Daiichi Sankyo's HER2 ADC 'Enhertu (trastuzumab deruxtecan)' in the new diagnostic classification of HER2 low breast cancer. However, there were also voices of criticism from some, concerned about whether the classification of HER2 low has any biological or clinical significance, and that it is ambiguous to distinguish it in the treatment field. Lunit has been observing the clinical results of Enhertu before, and at the same ASCO presentation site, Lunit first revealed the initial results of an AI-based HER2 analysis solution to overcome these diagnostic limitations.
The accumulated results of R&D over the past three years are coming out. As a starting point, Chan-young Ock, chief medical officer (CMO) of Lunit joined Lunit in September 2019, and the AI biomarker research that predicts the treatment response of cancer patients began in earnest.
In an interview with the biospectator, CMO Chan-Young Ock knew that immuno-oncology drugs are difficult to treat all patients and can only treat only part of the patients while being a professor at the hematologic oncology department of Seoul National University Hospital. There were needs on which patients to choose, he said. "I tried to solve the problem in various ways, including genomes, but it was not easy. In the meantime, I decided that it was a good way to see immune cells in the pathological image, and while listening to the introduction of AI technology from the members of the Lunit, I joined because I thought it would be fun to apply it in this direction."
He added, "It is important to treat patients in hospitals, but a way to make a difference in a larger area is to find new biomarkers, and I thought that biomarkers could help develop new drugs."
AI biomarkers, and the reason for pathology?
Considering the path he has taken so far, this decision may seem like an unexpected choice. CMO Ock is an oncologist and after obtaining a doctoral degree in translational medicine from Seoul National University, he has worked as a CMO in Medpacto and has experience in clinical development of TGF-β inhibitors. Since then, he has been a practicing professor in the Department of Hematology and Oncology, which prescribes medicines to patients. In that regard, it was interesting as to his thoughts on choosing pathology (cancer tissue) or diagnosis instead of the option of developing a new drug for combined administration of immuno-oncology.
CMO Ock said, “During research on cancer, the cause of cancer itself and all data are melted into the tissue. Since the early 2000s, researchers have been trying to understand cancer at the level of DNA and RNA genes. However, there was little need for biomarkers for cytotoxic anticancer drugs, and most targeted anticancer drugs were able to solve genomic approaches.” .
Then, with the advent of PD-1 and PD-L1 immunotherapy, predictive biomarker research was actively conducted to increase the drug response rate, and many pharmaceutical companies attempted to analyze the RNA profile expressed in real-time in the tumor microenvironment of cancer tissues. Representatively, MSD analyzed the gene expression profile (GEP) of 18 genes to develop a new biomarker to determine the extent of inflamed T cells in the tumor.
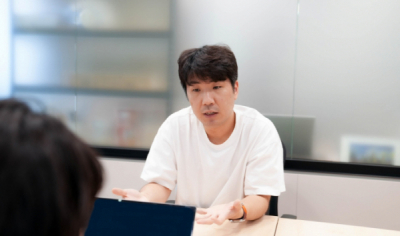
▲Chan-young Ock, chief medical officer
But every now and then they ran into failure. It is a logically good approach, but there were two major limitations. First, for practical reasons, it is difficult to obtain a sufficient amount of tissue in good condition to analyze RNA at the clinical site or clinical trial site. In this situation, RNA is degraded and information is damaged. Since there is a lot of RNA expression information, the standardization process is difficult. Second, immuno-oncology drugs must consider not only cancer cells in the tumor microenvironment but also the stroma surrounding them, but it is difficult to accurately read them unless you see them with your own eyes. At this stage, the weight is being placed on the fact that it is difficult to predict the response to immunotherapy with only RNA information.
CMO Ock said, “I thought that there was no way to solve the immunotherapy with the technology at the time, and a new direction was needed. As a more realistic approach, I thought that biomarker analysis should be performed at the tissue slide stage without damage after a fixation process in the pathology department. However, it is difficult for pathologists to understand objectively and comprehensively because of the vast and detailed image information of the pathology organization.”
Among these concerns, the keyword AI caught his eye. Since joining Lunit, he has increased the size of data to discover AI biomarkers in pathological organizations over the past three years, and has expanded data to multiple domestic and foreign institutions such as Northwestern University and Stanford University Hospital in the U.S.
Lunit is also speeding up its move to another axis, internally completing the immuno-oncology drug’s AI biomarker. In the past year, the AI biomarker project, which analyzes the prediction of drug response for target treatments such as HER2, has also been speeding up.
AI biomarker’s ‘immunotherapy’ platform
The Lunit SCOPE platform was first developed by focusing on immuno-oncology drugs. The most advanced project is Lunit SCOPE IO (immuno-oncology), which measures tumor-invasive lymphocyte (TIL) biomarkers in the tumor microenvironment of cancer tissue slides. Internally, these TIL biomarkers are judged to be 99% complete, and are in the final fine tuning stage.
Lunit SCOPE IO targets patients who have not previously been subjected to immunotherapy. It is a strategy to open the market by finding patients who can receive immunotherapy with Lunit SCOPE IO from cancers that cannot get prescribed for immune checkpoint inhibitors, or where they are approved but only prescribed to PD-L1-positive patients.
The mechanism is as follows. Basically, tumor infiltrating lymphocytes (TIL) in cancer tissues have a biological association with PD-L1. When an intratumoral mutation or a virus that induces tumorigenesis enters, it is a key signal transduction that raises the intratumoral interferon gamma signature (IFN-γ signature), resulting in increased PD-L1 expression and T cells expressing PD-1. flock to the tumor site. In addition, the overall PD-1/PD-L1 signature is increased, such as increased PD-L1 expression in macrophages and dendritic cells (DC). Accordingly, PD-L1 overexpression means that an intratumoral IFN-γ-mediated inflammatory response occurred, and T cell anticancer efficacy can be expected by inhibiting PD-1/PD-L1 signaling. Therefore, the higher the expression of PD-L1, the higher the probability that the TIL will also rise.
Nevertheless, the immune system is very complex, so PD-L1 does not accurately reflect the tumor microenvironment. “The most critical part is the stroma, and no matter how high the IFN-γ signature, it is difficult for T cells and tumor cells to interact spatially if there is a stromal barrier when found as immune desert or immune excluded in TIL analysis”, said CMO Ock.
In addition to IFN-γ signatures, PD-L1 may increase depending on the epithelial mesenchymal metastasis (EMT) mechanism that causes anticancer drug resistance, and the PD-1 treatment response rate may also decrease. This is also a case in which PD-L1 is highly expressed, but when TIL is analyzed, it is classified as immune deserted or immune excluded. Lunit wants to overcome these limitations by considering spatial information in analyzing TIL, and expects to play a complementary role since it approached from a different direction from PD-L1.
These ideas were reflected in the development process of Lunit SCOPE IO. AI biomarkers are created through two processes. First, an AI model that analyzes specific biomarkers in cancer tissue is created, and data values are put into the AI model created in this way to proceed with clinical demonstration.
Especially in the field of pathology, AI models are created, but the lack of standardized standards adds to the difficulty in development. CMO Ock said, "Relatively, it is not difficult to upgrade AI models to accurately read cancer or good/bad lesions because radiology platforms such as X-rays and CT, which have previously developed products, have already standardized data. But there are tens of thousands of cancer cells in the cancer slide, and the cancer tissue surface is rough."
Looking at the process of developing Lunit's AI model, the cancer tissue slide (H&E WSI) involves designating each point (annotation) as a data value according to the cell type (cancer cell/immune cell) and dividing (segmentation) the tissue type (cancer tissue/substrate) region. At this time, the accuracy is improved by discussing the results of each of the three pathologists reading the slides, and about 100 pathologists from home and abroad are participating in the development of Lunit SCOPE. After that, it will go through the process of training deep-learning-based AI models, with 40 to 50 internal employees.
Lunit SCOPE IO, which was created in this way, spatially analyzes TIL in cancer tissues and divides it into three types of IP: ▲inflamed immune, ▲ immune-exclusion, and ▲ immune-desert. It is predicted that the treatment response to immuno-cancer drugs will be different depending on the immune traits. Lunit has been publishing proof-of-concept results that confirmed clinical usefulness in a retrospective study method by applying Lunit SCOPE IO to existing clinical data.
As a major result, Lunit applied Lunit SCOPE IO to tissue samples of 518 non-small cell lung cancer (NSCLC) patients who received immune checkpoint inhibitors at JCO in March of this year and tested whether clinical benefit could be more accurately predicted according to immune traits. As a result of analyzing the dataset of patients receiving immune checkpoint inhibitor alone according to the immune trait classified by Lunit SCOPE IO, the overall response rate (ORR) , progression-free survival (PFS), and overall survival (OS) in the phenotype (inflamed immune phenotype, IIP) were confirmed to be statistically significantly improved compared to the other 2 types.
In order to find out that IIP+ is not a prognostic biomarker but a biomarker for predicting immune checkpoint inhibitors, a dataset of patients who received platinum-based chemo-cancer drugs alone in the first treatment setting was analyzed. This is because IIP+ can be interpreted as a prognostic biomarker if the clinical advantage varies depending on the immune type even in patients who have been given only chemotherapy drugs. As a result of the analysis, there were no ORR and PFS differences in the chemo-cancer drug administration group, and OS indicators showed that the IIP+ group tended to be numerically long, but no statistical significance (36.2 vs 22.9 vs 20.9 months). In other words, it is data showing the possibility that Lunit's TIL biomarker will serve as a biomarker predicting the benefits of immunotherapy rather than a prognostic biomarker.
▲JCO key result
The research team even looked at whether the TIL biomarker could complement the existing PD-L1. As for the score that measures PD-L1 expression (TPS), more than 50% predicts an immune checkpoint inhibitor response well, but there is a limitation in that the accuracy of predicting drug response is poor in the TPS 1-49% group with relatively low expression. Accordingly, in 1-49% of TPS, it was evaluated whether TIL biomarkers could better predict clinical benefit. As a result, when the TIL biomarker was applied to the TPS 1-49% group where PD-L1 did not perform well, it was confirmed that the IPP+ group predicted PFS better than other immune-desert/excluded groups (4.0 vs 2.1 months, HR=0.54, p=0.001). In addition, it was confirmed that Lunit SCOP IO had higher predictive accuracy (AUROC) for immune response compared to PD-L1 in the TPS 1-49% group (0.7609 vs 0.5561, p<0.05). However, there was no difference according to the immune trait in the OS index.
Lunit took one step further from this result and saw the possibility of expanding indications. The possibility of applying the TIL biomarker to all solid carcinomas beyond lung cancer was demonstrated. As a result of the results presented at this year's ASCO 2022, tissue samples (17,849) of 1,806 cancer patients across 16 carcinomas were analyzed with Lunit SCOPE IO. As a result, there was a significant difference between the IIP+ and IIP- groups in ORR index (26.0% vs 15.8). %, p<0.001). In the PFS index, it was also found that the IIP+ group lowered the risk of stage progression or death by 34% compared to IIP- (9.0 vs 5.3 months, HR=0.68, p<0.001). In addition, excluding non-small cell lung cancer (909 patients) patients from the overall data, there was still a clinical benefit.
▲ASCO 2022
As a result, Lunit SCOPE IO is being developed as a product to find patients who can respond to immunotherapy regardless of PD-L1 by spatially analyzing TIL in cancer tissues. “Lunit SCOPE IO is intended to be used as a biomarker that makes an appropriate prediction in all carcinomas regardless of carcinoma, and when developing AI models, it has been optimized to have consistent accuracy in each carcinoma.” said CMO Ock.
It is also preparing a prospective clinical trial to develop an immuno-cancer drug companion diagnosis (CDx) product, and is discussing this with the FDA. Prospective clinical practice refers to testing drugs only on positive patients according to the determination of biomarkers from the patient screening stage, and regulators require a higher level of evidence. So far, there have been no cases of using AI biomarkers as assistive or companion diagnostic biomarkers in clinical trials for new drugs.
Expansion is also expected in the diagnosis area. Lunit believes that TIL biomarkers can be applied not only to the single administration of immuno-cancer drugs but also to PD-(L)1 combined administration. According to a study published in Clinical Cancer Research this month, the IIP+ group confirmed the improvement of PFS when TIL biomarkers were applied to tissues of nasopharyngeal cancer patients who were given Opdivo and chemotherapy drug "Gemcitabin." Nasopharyngeal cancer is a cancer in which PD-L1 does not function as a biomarker, and is a result of a study showing the possibility of applying TIL biomarkers.
It is expected that it can be applied to improve the treatment policy of immuno-cancer drugs in the future. If PD-L1 is expressed more than 50% in non-small cell lung cancer, there is an option for combination administration of Keytruda or Keytruda + chemo drugs. Adding chemo drugs to PD-1 antibodies increases the number of patients who can be treated, but there is a concern that the treatment period may be reduced due to toxicity. Therefore, it is an approach to select patients who do not need to be prescribed for chemo drugs with TIL biomarkers. It is the same concept as Oncotype DX, which predicts the prognosis of breast cancer and decides whether to treat chemotherapy after surgery.
Expansion to targeted therapy platform..7 projects in progress
Another area that Lunit is focusing on in the future following AI biomarkers for predicting immunotherapy is the field of targeted therapy drugs. We are focusing on the development of AI models that quantitatively measure cell membrane proteins seen in immunohistochemistry (IHC) slides. Seven projects are underway, including Lunit SCOPE HER2.
“Recently, targeted therapy technology such as ADC, CAR-T, and T cell engager has been growing rapidly,” said CMO Ock. “As a result, the importance of accurately evaluating the expression of specific biomarkers in the patient's tissues is growing. We expect it to become as important as the Lunit SCOPE immuno-cancer drug area in the future."
Lunit has a team that tracks Big Pharma's clinical results from a team that understands industry trends inside, so it is selecting promising targets six months to a year ahead. Currently, it takes three to four months to develop the AI model for a targeted treatment, and it is expected that the speed will gradually decrease as data and know-how accumulate. Lunit SCOPE HER2, which was first unveiled by Lunit as a targeted cancer drugs AI platform, also expected ADC to produce positive results at HER2 low, and it was able to show data at ASCO by speeding up its development from the beginning of this year.
AstraZeneca newly defined HER2 low patients in ASCO 2022, administered Enhertu to HER2 low metastatic breast cancer patients, halving the patient's risk of stage progression or death compared to existing treatments, and improving the overall survival period (OS) by more than 6 months. As a result, Enhertu redefined the HER2 market, receiving approval for marketing licenses 11 days after entering the new drug review. In addition, previously, patients who could receive HER2 targeted treatments were 10-15% positive for HER2, but it expanded to about 60-75% as HER2 low was included with Enhertu’s marketing authorization.
HER2 low, which is now subject to Enhertu prescription, is a new type that has been previously determined to be HER2 negative. Specifically, it means IHC1+ or IHC2+/ISH-, accounting for about 50% of all breast cancer patients. HER2 positive is defined as IHC3+ or IHC2+/ISH+ and corresponds to about 15% of all breast cancer patients.
The problem is that although the HER2 IHC3+ or IHC0 pathologist reading agreement rate is high, the IHC1+ or IHC2+ reading belonging to HER2 Low has a high interobserver variation. In other words, HER2 low still differs depending on the pathologist's diagnosis, and there is a concern that the patient may not receive appropriate treatment.
To overcome these limitations, Lunit reads the degree of HER2 expression as a specific number such as '1.7, or 1.03' for those between 1 and 2. It is explained that the accuracy can be increased by measuring HER2 expressed in cancer cells and excluding cases expressed in other tissues. In the future, AI expects to find additional patients in patients identified as HER2 negative because it can even capture detailed expressions from tissue images.
According to the results released by Lunit at ASCO this year, if pathologists use AI models to read HER2 IHC results from breast cancer tissues, they can increase the reading matching rate among pathologists. As a result, the concept is that applying Lunit SCOPE HER2 allows targeted treatments such as Enhertu to find an accurate curable cut-off and expand treatable patients.
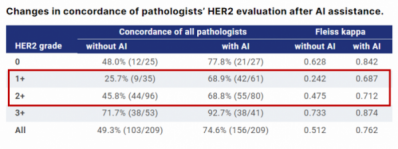
▲ASCO 2022
So, what is the competitive advantage compared to global AI biomarker developers? The difference between Lunit is ‘selection and concentration’ and ‘data presentation’ are the keywords.
He explained that creating an AI biomarker platform in the field of pathology requires selection and concentration, and that pharmaceutical companies are not at the level of proceeding in parallel while developing new drugs. In the field of pathology, Big Pharma has abundant internal clinical pathology data, so it may seem advantageous to proceed with direct development, but it is not. It is similar to using Lunit INSIGHT solution as a tool for global medical device companies such as GE Healthcare, Fujifilm, and Philips to analyze photographic images with devices.
CMO Ock explained, "In a short example, 16,000 slides were used to develop Lunit Scope IO, but only 150 AI models made by pharmaceutical companies were learned," adding, "We believe that Lunit's ideas on how to create AI models, such as data size and annotation methods, are three to four years ahead of this big pharma."
Another strength of Lunit is the presentation of data. So far, more than 150 cases have been published at the conference, and more than 10 SCI-level papers (IF 10 points or more) have been published. This year, 11 poster presentations were held at ASCO alone. This is a significantly higher number compared to competitors PathAI and Owkin.
CMO Ock said, "Lunit is rapidly accumulating clinical evidence as well as technology," adding, "Big Pharma, which has discussed with its competitors as a positive part, has been continuously contacting them in the past year." I think the game will change a lot in a year or two," he said.
▲Competitive landscap
Global commercialization partner, Guardant Health
A commercial partner for the pathology AI biomarker is Guardant Health (GH), the leading US precision medicine liquid biopsy company. In July last year, Lunit received an investment of 30 billion won from GH and signed a strategic partnership for the Lunit SCOPE model. At that time, it became a hot topic in the industry as it was the first investment since its establishment in 2011.
CMO Ock said, “Guardant Health has a central lab, so it has the advantage of being able to standardize the process of tissue processing, staining and scanning well. Through the CLIA lab verification, it was decided that the Lunit SCOPE IO results analyzed in the laboratory could come out as a consistent result.”
Lunit have already received milestones as they have completed the development of two Lunit SCOPE models for use in the CLIA lab at GH. After that, if the AI model is installed in the GH product, it will receive an additional milestone of success. CMO Ock said, "We are developing and preparing to release AI models such as Unit SCOPE IO, PD-L1, HER2, ER, and PR, so we expect tangible results within this year."
In the future, it plans to advance discussions with global pharmaceutical companies and biotech companies through GH. It is currently discussing the application of various assets from preclinical to early and late clinical stages.
Lunit's partnership with GH will also see its scalability to early diagnosis areas in the future. GH is expanding its early diagnosis portfolio such as colon cancer with genetic information through liquid biopsy. Lunit has published results in mammography images that the Lunit INSIGHT MMG solution not only catches breast cancer lesions, but also distinguishes high-risk groups at risk of developing breast cancer within the next one to three years in patients who are determined to be normal. CMO Ock added, "We believe that the liquid biopsy platform of GH and the method of finding high-risk patients in Lunit's imaging diagnosis solution can be complementary and become an early diagnosis model."
AI biomarker 'CDx target'...a long-term vision
About the milestones that will be visible in the next year or two. CMO Ock explained, "It is important to accumulate data showing the clinical benefits of AI biomarker models in the short term, and we plan to present the AI biomarker platform for targeted anticancer drugs at various conferences."
Based on the accumulated data, the goal is to prove that Lunit's AI biomarker predicts the treatment response of drugs such as immuno-cancer drugs and targeted therapy drugs through partnerships with pharmaceutical companies and Biotech, and to release CDx products in the mid to long term. In the field of AI biomarkers, models such as Foundation Medicine and Guardant Health, which are representative companies in the field of therapeutic agent companion diagnosis.
Several business development models are also being devised. New AI biomarkers can be discovered to increase the success rate of clinical development of new drug candidates, and risk sharing through strategic investment (SI) in Asset can be considered, and drug licensees can be considered in the long run.
CMO Ock said, "Lunit has a vision to conquer cancer, and I think it can provide directions from early diagnosis to precision medicine," adding, "We have started genetic research internally, and we will expand various modalities because AI technologies can be applied to medicine."
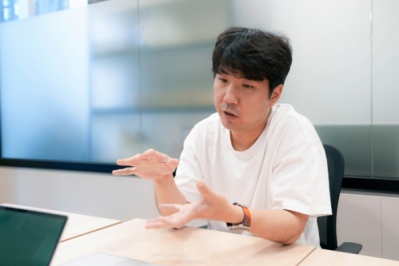
▲Chan-young Ock, chief medical officer